The Italian EAGE-SEG Section interviewed Massimo Fervari about the current applications and future perspectives on Seismic Inversion techniques, a technical topic with enormous implications on the E&P activities of Energy Companies. Seismic Inversion has several applications and Massimo’s brain is certainly among the most passionate ones to pick.
What are the main Seismic Inversion methodologies and techniques that are implemented in Eni’s industrial processes?
At Eni, the process of inversion and quantitative description of the reservoir properties is rooted in the late 1980s, with experiments on 2D data and the first 3D volumes. It still represents the core of Seismic Reservoir Characterization activities.
The SRC team supports
- exploration teams for:
- “Litho-fluid de-risking”;
- “Prospect assessment”;
- “Reservoir delineation” in the appraisal phases.
- development teams for:
- “Construction” of the reservoir model and its continuous updating,
- “Monitoring” of Production activities and operational phases of execution;
- “De-risking”;
- “Optimization” of the trajectories of the development and infilling wells.
Seismic Inversion methodologies are based on a wide range of different workflows and algorithms, which combine seismic data (pre-stack, partial stack or full stack) sequentially or simultaneously. Seismic inversion methods can be organised into three large groups:
- deterministic approaches: the more conventional ones, which include acoustic or elastic solutions and for example Model Based or Sparse Spike Inversion approaches (algorithmic part);
- probabilistic approaches, which use Bayesian approach to measure the uncertainty associated with the most probable model;
- geostatistical approaches, which combine previous techniques with well information (through its variance trend) to increase the resolving power of the modelled data, using at the same time more advanced sampling approaches of probability distribution (i.e. Monte Carlo Markov Chain – MCMC algorithms) looking for complex objective functions in nonlinear inverse problems.
Classically, the deterministic approach is composed by two steps:
- Transformation of seismic data into elastic parameters;
- Calibration and classification of elastic parameters of the reservoir (including facies) or lithology/fluid indicators, using different technologies (statistics, probabilistic, neuronal, etc.).
Relating to probabilistic approaches, “multi-step” and “single loop” workflows are available to derive litho-petrophysical properties and facies model.
This approach assimilates the uncertainties measured at each step and provides an elastic and a posterior, conditioned, petrophysical medium model (or facies) with associated uncertainty for each variable.
Geostatistical approaches are also available, which generate equally likely realizations of elastic parameters and facies. They can be used individually or globally to define desired scenarios, through the ranking of specific variables (i.e. Net / Gross, porosity, etc.), conditioning the reservoir modelling and directing the model uncertainty analysis.
In some cases, the stack of equi-probable realizations can allow the return to a pseudo-probabilistic facies framework, which preserves a greater vertical resolution, usable as a seismic trend in the model.
In addition, there are many other methods of seismic inversion:
- Extended Elastic Impedance;
- Gradient Impedance;
- Rotational approaches of inverted elastic properties, which optimize the description of litho-petrophysical components and fluid properties. These factors support, with other AVO technologies, exploratory de-risking and seismic-quantitative analysis for fluid prediction.
Also, seismic inversion methods support 4D and Time Lapse studies, for reservoir monitoring projects during the production phase.
In these projects, the inverted elastic parameters are used quantitatively to integrate the analysis of seismic amplitudes to implement simultaneous inversion protocols, overcoming the classical sequential approaches, which evaluate the differences between baseline and monitoring data.
Beyond the algorithms and technical inversion procedures in the strict sense, in Eni great emphasis has been given to the integration of the Rock Physics component, to optimize the available well data.
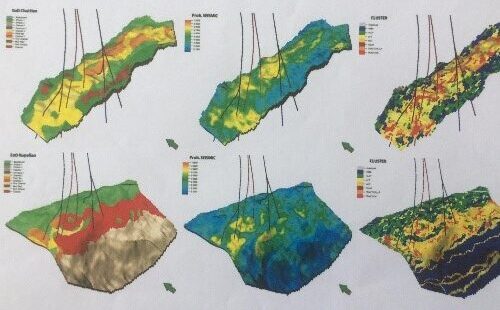
Figure 1 – West Africa Deep Water: integration of Inversion results whitin the Reservoir Modeling Workflow. (previously Published on First Break)
The SRC team evaluates many scenarios not represented by well data such as prior geological information, which can be used in inversion and calibration of the parameters, in order to obtain a truly robust quantitative geophysical model.
The final aim is the best reconstruction and description of the geological model of the sub-surface, at different scales, supporting diversified activities along the exploration and development cycle.
From your point of view, what are the objectives that industrial research should pursue in the near future?
Many technological improvements can be made:
- Development and implementation of new algorithms:
- Rock Physics modelling should integrate the formulation of the objective function;
- The use of Bayeasian approaches should enhance. They produce reverse solutions with associated uncertainty;
- In the process the possibility of using many constraints (especially geological), which condition the model with all the information available. Therefore, it could be reduced the space of the infinite possible solutions, mitigating the issue of the non-uniqueness of the inverse solution.
- Improvement of multi-dimensional integrated workflow:
- The integration between the Petrophysical model and the Rock Physics model, aims to define a petro-elastic frame, which is functional to generate an optimized facies model. Facies modelling is useful to predict reservoir properties, starting from seismic data. It is also useful for reservoir modelling to capture and to describe the physical heterogeneities, which actually influence the knowledge of static and dynamic processes.
- The definition of advanced approaches for the construction of the Low Frequency Model. At Eni, it is often based on iterative multi-loop workflows, which integrate interpretative processes (detection of geological features), well information and seismic velocity models, generally using depth imaging. Geological information is used as an indicator of the burial history; they capture the main elastic trends associated with the phenomena of compaction.
- Another important aspect is the integration of the stratigraphic-depositional model in the seismic space of the inversion model, not only conceptually, but allowing on a different scale the quantitative use of prior information (i.e. the expected facies probability) to influence the result of the inversion with information already available.
- The optimization of the velocity field used for the conversion of seismic properties into the depth domain. On this aspect, to obtain the best depth matching of the derived properties with all the available geological information, workflows have been implemented in the structural model framework. These workflows integrate the calibrated PSDM velocity field with the inversion result, to improve the positioning depth of intra-reservoir events not directly interpreted.
- The use of the reservoir properties (i.e. facies or petrophysical grids) to optimize the reservoir model, through conditioned geostatistical inversion approaches. Otherwise, this optimization could update the reservoir model using new information.
- Focusing on the geostatistical approaches, which are already available in reservoir modelling tools. These tools should allow to treat elastic variables spatially with the same flexibility used in reservoir modelling, increasing at the same time the resolution of the (conditional) inversion model, making it more usable in the static modelling workflow.
- Opening new fronts of integration between seismic inversion approaches, with associated uncertainty, and static-dynamic modelling, assimilating the geophysical results (3D and 4D) in fully integrated workflows, to support the process of “ensemble- based” history matching. Optimal interpretation takes place in these workflows of the production data and the possible updating of the static-dynamic properties, maintaining at the same time the process efficiency and the consistency of the G&G information related to the static modelling.
- Anisotropic inversion will be implemented in the future: VTI and HTI approaches will have a privileged development line, integrating multi-azimuth approaches; moreover:
- the inversion on 3D VSP and 4D VSP data (with DAS technologies), on which recent successful experiments have also been carried out by Eni;
- the increasing use of Frequency information in the AVO inversion of pre-stack data: today it is not fully integrated into structured workflows;
- the application of multi-component inversion methodologies (PP-PS), often limited by the scarce availability of an adequate seismic starting data.

Figure 2: Middle East/Carbonate Field: Porosity Volume after Seismic Inversion. (previously Published on First Break)
What impact did the new technologies have on the industrial operational processes of Seismic Inversion (Machine Learning, Big Data, Data Management, Artificial Intelligence, Coding, High Performance Computing, Neural Network…)?
Regarding artificial intelligence, it is not always easy to define a precise relationship between processes supported by fundamental physical principles (Theory-based models or Physics-based modelling, generally based on laws defined by a limited amount of information) and mathematical-statistical approaches (data-driven models) based on self-learning algorithms on large amounts of data.
Anyway, I believe that future developments will lead us to develop hybrid solutions (Theory-guided data science models), to exploit the advantages of new technology, without ignoring the value of the knowledge accumulated by the scientific principles acquired. This is the direction Eni is moving towards.
In the specific case of Machine Learning applications to seismic inversion-calibration processes, some preferential directions can be outlined:
- Identify the artificial intelligence workflows which can integrate the physical inversion-calibration process, for example:
- Generation of pseudo-wells, which are supported by Rock Physics modelling. They could analyse the relationship between seismic data and well data, supporting the estimation of the wavelet and its uncertainty.
- Evaluation of the “a priori information”, applying unsupervised and supervised classification techniques on inverted data, aimed to classifying the reservoir properties and the organization of data in clusters, facies, etc.
- Machine Learning process could “physically assist” the generation of training dataset, to enforce Deep Learning analysis. There are many research initiatives in which, through the models of Rock Physics and quantitative seismic (forward and inverse modelling), training datasets are generated based on Input-Output pairs used in the analysis of non-linear relationships through Deep Learning processes. They can be applicable for the generation of elastic parameters or directly related to lithological-petrophysical properties, replacing the traditional inversion.
New digital technologies have then given a big impulse to include more and more the uncertainty. Indeed, the inversion process only provides approximate images of the sub-surface, not exact images: it is therefore correct that the deterministic solution is replaced by a set of compatible solutions, with different probabilities or from families of equi-probable realizations.
From this point of view, the digitization process and the possibility of analysing large amounts of information in even shorter times (referring to Big Data), should strongly encourage this direction of future development.
What do you think could be the future developments and opportunities of Seismic Inversion in the context of the energy transition?
In the context of energy transition, all technologies will take on great importance, which will be able to ensure the necessary support for the faithful reconstruction of the sub-surface model, an essential knowledge for any type of application: the quantitative seismic methodologies are obviously placed in this context, including seismic inversion workflows and elastic, lithological, petrophysical characterization, which relate to the fluids contained in the rocks.
A particular importance will be given to the support of the geomechanical model, (already done by Eni), integrating it with the structural model. They are the new protagonists of projects with high environmental sustainability, guiding quantitative techniques, previously oriented to the reservoir alone, towards the characterization of the entire overburden sequence, in integration with an appropriate development of Rock Physics models for non-reservoirs, increasingly supported by experimental data acquired in the laboratory.
The development of new seismic inversion parameters (i.e. compressibility parameters of the rock) will be able to support better the quantitative assessment of injected gas. This is very important for the monitoring of CO2 injection in Carbon Storage projects.
What advice would you give to a young geophysics student who has the desire to work in the context of Seismic Inversion?
Seismic inversion remains for me a very fascinating topic, even after a long personal experience of thirty years spent in the field. The continuous technical development requires, as in other technical-scientific fields, updating continuous knowledge and the adoption of different methodologies, increasingly integrated with geological background.
Then, I would suggest to our young colleague to continue his studies by balancing the physical-mathematical-statistical knowledge with an in-depth and not superficial knowledge of the geological phenomena and of the sedimentary record, at the basis of each subsequent application.
If you went back to being a student for a moment, what would you have like to have known to face your career in a better way?
First of all, I would have organised a course of study supported by adequate technical knowledge and specific advising, which would have greatly facilitated personal development, which a few decades ago, with some exceptions, did not represent our average background.
Anyway today, in the era of hyper-communication there is no lack of information, there are many opportunities to deepen the study subjects with specific masters and internships in industrial companies. A good initial background is certainly an excellent business card to undertake our business in Energy Companies. There are many opportunities in the academic field, although generally the Italian reality remains rather closed and complex, especially abroad as demonstrated by our excellence distributed throughout the world.
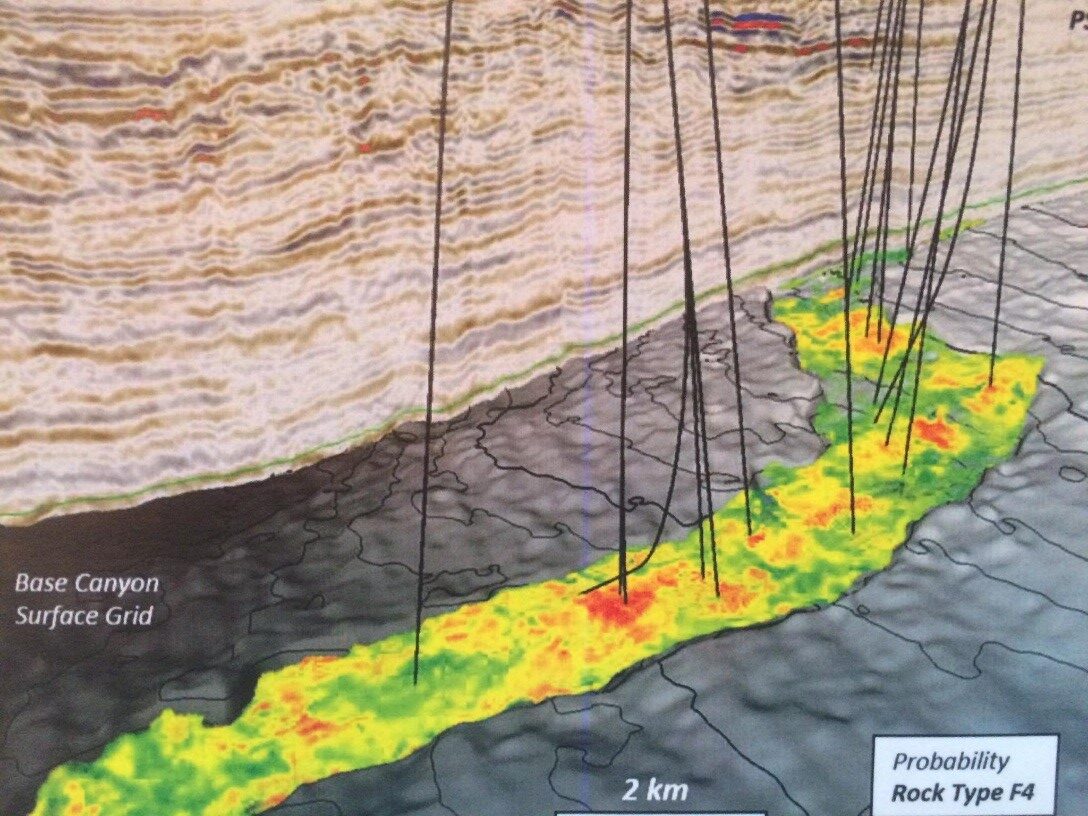
Figure 3: West Africa, Deep Water: Facies Probability Volume. (previously Published on First Break)
What would today’s professional say to the student of that time after your professional experience?
After having discussed the importance of knowing in depth the technical aspects related to seismic inversion processes, I would close this interesting dialogue by suggesting to students and young colleagues that knowing and knowing how to do is not enough.
Apart the importance that soft skills now play in team dynamics, especially those of communication and cooperation for the achievement of common goals, I believe that the key to personal success lies in having passion! We will be able to say that we have been successful in our professional career if after a long campaign of work commitment and research we are moved by the same initial enthusiasm … This is perhaps my best wish for every young person who today follows new path.
Recent Comments